Interview with Lukas Vermeer, Data Scientist, Booking.com
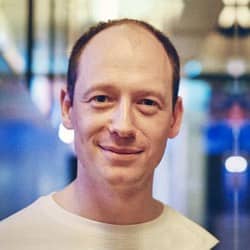
Lukas Vermeer, Data Scientist,
Booking.com
True innovation starts with asking Big Questions.
Hi Lukas, you are a data scientist at booking.com, the world’s leading accommodation website. Please tell us, how is booking.com using predictive analytics and what is your role as data scientist at booking.com?
Booking uses predictive analytics for lots of different things! In web marketing, attribution models and ROI predictions help bring customers to our site. On the product side, recommendation systems help us show more relevant destinations, hotels and content to our users. In customer service, call volume predictions and scheduling algorithms help staff our call centers and connect customers to the right agent as quickly as possible. I could go on. In fact, I honestly struggle to think of a single department that is not using predictive analytics in one way or another.
My personal experience at Booking started on the product side, where I worked on improving our recommendation and ranking algorithms. After that, I’ve specialised in the design of controlled experiments and hypothesis testing for product development. Validating that changes to our product have the expected impact on customers and partners is an important part of our product development cycle. Unlike many other companies, we try to validate almost all changes we make to our product through in vivo randomised controlled trials. To achieve this, we run experiments at a massive scale. We need all of our product development teams to understand the basics of hypothesis testing and the statistics behind it. My job is to improve the tooling and education that makes this possible.
Your keynote at the upcoming Predictive Analytics World Business in October in London will be about “Data Alchemy” – what’s data alchemy and what will your presentation cover?
In my opinion the “Big Data” and “Data Science” rhetoric of recent years has been focused too much on collecting, storing and analysing existing data. Data which many seem to think they have “too much of” already. However, the greatest discoveries in both science and business rarely come from analysing things that are already there. True innovation starts with asking Big Questions. Only then does it become apparent which data is needed to find the answers we seek.
I use the term “data alchemy”, as opposed to “data science”, to describe this misguided focus on analysing and collecting existing data. Science is about asking important questions and thinking about what data would need to be gathered to answer them. Alchemy on the other hand was (largely) about trying to turn lead into gold. When people ask me how to “extract useful information from all this data that we have” I tell them they need a data alchemist, not a scientist.
Ok, so you are saying the data scientists should not only think about how to analyse existing data but also ask themselves how to find new sources of data that helps them to build better predictive models. Can you give us a few practical examples of how to achieve this challenge of finding or creating new data sources?
Yes. I think in many cases it is much easier to improve model performance by changing the way in which data is collected then by changing the way it is processed. I’ll give a few examples of this in the talk.
What is more, when building products using predictive analytics, we should also think about how our models will affect the data we will have available going forward. Take recommendations systems as an example. The recommendations such a system gives will (hopefully) influence what products people see and buy. Most likely, that behavioural data will in turn be used to build the next generation of models. That means our recommendations have two effects: on immediate user behaviour, but also on future model performance. As data scientists, we need to understand that what we build will influence the world in ways that might affect the data we collect, and how we can use this to our advantage.
A critical part seems to be the cultural change within the companies: data scientists have to talk and interact with the software developers, the marketing experts etc. to create products and campaigns that gather the right data for predictive models. Is this something an average data scientist could carry out successfully or do companies need additional roles, such as data strategists and data stewards?
I’m not sure this is something that could be solved by adding more roles. I think that in general many companies need to rethink their organisational silos and approach to product development. Teams should be centred around an objective or product, not around a set of skills. That way, data scientists, developers and marketing experts can work together much more closely in heterogeneous teams to achieve company objectives, such as building better predictive models.
I realise that this is an unconventional way of structuring an organisation, but it makes so much more sense then having separated IT, Marketing and Sales departments struggling to build a product which none of them fully owns. For me, it was one of the main reasons to join Booking.com. Working in small teams with mixed skills to achieve a clear task gives me a sense of ownership, purpose and empowerment that I was missing when working in other large organisations.
Last question for today: what do you think about the current awakening of artificial intelligence? Are we heading for an AI summer – or will there be a new AI winter?
I can build predictive models, but I don’t think anyone can really predict the answer to your question. There’s a lot of hype, but I also see a lot of progress. I’m sure there will be another winter at some point, as many people will undoubtedly be disappointed by the limitations, but for now things look sunny.
Thanks for the interview, Lukas! We are looking forward to your keynote at the Predictive Analytics World London!
July 19, 2017